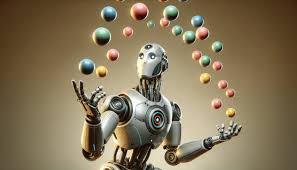
Artificial Intelligence (AI) is undeniably a transformative force, influencing industries from healthcare to finance, and from education to entertainment. Yet, despite its remarkable capabilities, AI continues to face a significant trust deficit. This skepticism arises from a set of persistent risks that have undermined public confidence. Understanding these risks is essential for addressing them effectively and fostering greater trust in AI systems.
1. Bias in AI Systems: One of the most glaring issues in AI is the presence of bias. Since AI systems are trained on historical data, they can inadvertently inherit and amplify existing societal biases. For example, algorithms used in hiring processes have been found to discriminate against certain demographics based on gender or ethnicity. Such biases not only lead to unfair outcomes but also erode trust in AI as an objective decision-making tool.
2. Transparency Challenges: AI systems, especially those utilizing deep learning, often operate as “black boxes,” where their decision-making processes are not fully understood, even by their creators. This lack of clarity makes it challenging for users to comprehend how outcomes are derived. In sensitive areas like healthcare diagnosis or judicial verdicts, this obscurity can lead to concerns about accountability and erode trust in the technology.
3. Concerns Over Data Privacy: AI depends on extensive datasets to function efficiently. However, gathering and utilizing personal data raise serious privacy issues. Cases of unauthorized access, data breaches, and invasive surveillance have amplified fears about potential misuse of personal information by AI systems. Without strong protective measures in place, these privacy concerns are likely to fuel continued skepticism and hesitation regarding AI adoption.
4. Security Vulnerabilities: AI systems are susceptible to cyberattacks, making security a significant concern. One prominent threat is adversarial attacks, where attackers intentionally modify input data to mislead AI systems. For example, even slight alterations to an image, such as changing a few pixels, can cause the AI to incorrectly identify it. These weaknesses highlight critical issues regarding the safety and dependability of AI technologies.
5. Ethical Dilemmas: AI introduces complex ethical challenges. Autonomous vehicles, for example, may face situations where they must make life-and-death decisions, raising questions about whose lives should be prioritized. Similarly, the use of AI in warfare and surveillance has sparked debates about its potential misuse. These ethical concerns contribute to the growing mistrust of AI systems.
6. Economic Displacement: AI’s potential to automate jobs has raised fears about economic displacement and inequality. While AI promises increased efficiency, it also threatens to render many jobs obsolete, particularly in industries reliant on routine tasks. The lack of clear policies to address these disruptions exacerbates public anxiety and skepticism about AI’s role in society.
7. Over-reliance on AI: As AI becomes increasingly integrated into critical systems, there is a risk of over-reliance. Blindly trusting AI without adequate human oversight can lead to catastrophic outcomes. For example, financial institutions relying solely on AI for trading decisions have experienced significant losses due to unforeseen market anomalies. Trust must be balanced with caution.
8. Accountability and Legal Challenges: When AI systems fail or cause harm, determining accountability can be challenging. Is the blame on the developers, the users, or the AI itself? The lack of clear legal frameworks for addressing such scenarios creates uncertainty and fuels mistrust. Establishing accountability mechanisms is crucial for fostering confidence in AI.
9. Misinformation and Deepfakes: AI technologies, particularly generative AI, have made it easier to create convincing fake content, such as deepfake videos and synthetic news. These tools can be weaponized to spread misinformation, manipulate public opinion, or harm reputations. The potential for misuse of AI in this context has become a significant concern.
10. Lack of Inclusivity: AI development has often been concentrated in a few regions and controlled by a handful of corporations, leading to a lack of diversity in perspectives. This centralization can result in technologies that do not adequately address the needs of underrepresented communities. Ensuring inclusivity in AI development is essential for building trust.
11. Environmental Impact: The computational power required to train advanced AI models has a significant environmental footprint. For instance, training large language models consumes vast amounts of energy, contributing to carbon emissions. This environmental cost has led to criticisms of AI’s sustainability and its alignment with global climate goals.
12. Unrealistic Expectations: AI is often marketed as a solution to all problems, leading to inflated expectations. When these expectations are not met, disillusionment sets in, eroding trust in the technology. A more realistic portrayal of AI’s capabilities and limitations is necessary to build long-term confidence.
Building Trust in AI
Effectively tackling these twelve ongoing risks demands collaborative efforts from all key stakeholders, including developers, policymakers, and users. The following strategies can help in restoring and strengthening trust:
- Enhancing Transparency: Developing explainable AI models that allow users to understand how decisions are made is crucial. Providing clear documentation and open-source solutions can also contribute to transparency.
- Mitigating Bias: Regular audits and diverse training datasets can help reduce bias in AI systems. Involving ethicists and domain experts in the development process can ensure more equitable outcomes.
- Strengthening Data Privacy: Implementing stringent data protection measures and adhering to regulations like GDPR can reassure users about the safety of their data.
- Strengthening Security Measures: Allocating resources to research focused on identifying and mitigating adversarial threats is crucial. Conducting routine security evaluations plays a key role in uncovering potential weaknesses and addressing them proactively.
- Â Promoting Ethical Practices: Establishing ethical guidelines and oversight committees can address moral dilemmas associated with AI. Encouraging public dialogue on these issues can also foster greater understanding.
- Supporting Workforce Transition: Governments and organizations must invest in reskilling programs to prepare workers for jobs in an AI-driven economy. Social safety nets can help mitigate the economic impact of displacement.
- Balancing Human-AI Collaboration: Ensuring human oversight in critical AI applications can prevent over-reliance. Hybrid decision-making systems that combine human judgment with AI capabilities can enhance reliability.
- Defining Legal Boundaries: It is essential for governments to implement well-defined regulations to ensure accountability in the use of AI systems. This involves specifying responsibility and liability in scenarios where AI systems cause harm.
- Combatting Misinformation: Developing AI tools to detect and counter fake content can mitigate the risks posed by deepfakes and misinformation. Public awareness campaigns can also help users identify and avoid false information.
- Fostering Inclusivity: Promoting diverse teams in AI development and involving local communities can ensure that AI technologies address a broader range of needs and perspectives.
- Reducing Environmental Impact: Prioritizing energy-efficient AI solutions and taking steps to offset carbon emissions can help minimize the ecological effects of AI. Partnerships between technology companies and environmental groups play a crucial role in achieving this goal.
- Managing Expectations: Providing accurate information about AI’s capabilities and limitations can help align public perceptions with reality, preventing disappointment and fostering trust.
Conclusion
AI’s trust problem is not insurmountable. By addressing the risks outlined above, stakeholders can pave the way for a future where AI is not only powerful but also reliable, ethical, and inclusive. Building trust in AI will require transparency, accountability, and a commitment to aligning AI’s development with societal values. Only then can AI truly fulfill its promise as a force for good.
See More:
[…] AI Trust Challenges and Solutions […]